IASP's 50th Anniversary: Working Together for Pain Relief Throughout the World.
IASP condemns the pain and suffering caused by the violence of war, especially to civilian populations and hospital facilities. We are grateful for the health care workers, physicians, and scientists working hard to relieve pain in difficult and dangerous circumstances.
IASP 2024 World Congress on Pain
5 - 9 August I Amsterdam, Netherlands
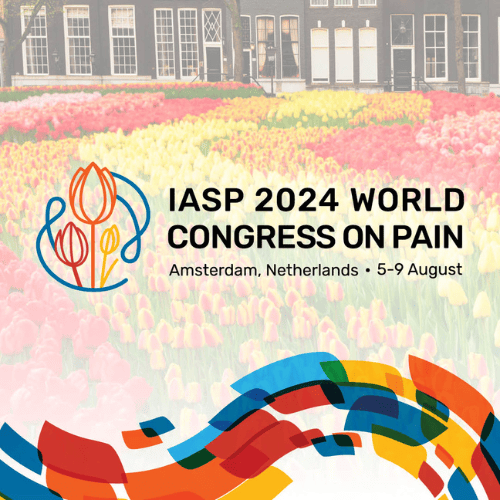
IASP’s World Congress on Pain is the largest gathering of pain professionals in the world, bringing together more than 5,000 scientists, clinicians, and healthcare providers from around the world and across pain disciplines. Attendees will experience a world-class lineup of more than 400 international speakers with comprehensive sessions bringing you the latest pain research and treatment. Join your fellow pain professionals for:
- 2,500+ poster abstract presentations
- 100+ Exhibitors
- 93 Topical Workshops
- 33 Pre-Congress Events
- 10 Plenary Lectures
- 2 Nobel Laureate Keynote Lectures
The World Congress will include the IASP 50th Anniversary celebration with reflections on industry advances in pain research and IASP milestones over the last 50 years. Join IASP 5 – 9 August in Amsterdam to commemorate 50 years of working together for pain relief throughout the World.
Upcoming Events
Pain Journal
PAIN
The official journal of IASP features original research on the nature, mechanisms, and treatment of pain.
Pain Reports
GLOBAL YEAR
IASP 2023 Global Year for Integrative Pain Care
Global Year aims to increase awareness of pain knowledge and how it may benefit those living with pain.
Pain Research Forum
Pain Research Forum
Pain Research Forum (PRF) is the premier destination for pain research. We translate new knowledge of pain into new treatments for pain conditions. Explore the latest research findings and news, thought leadership, and papers of the week below.
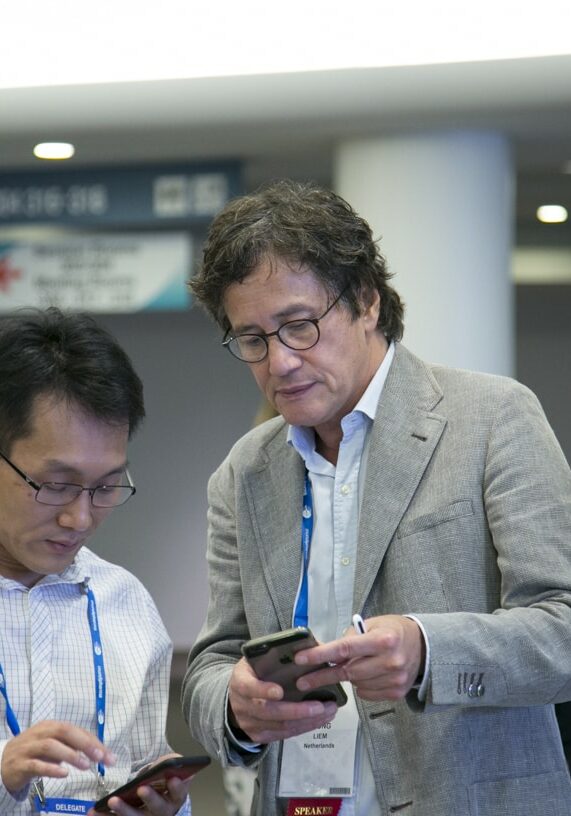

RELIEF NEWS
Relief News
RELIEF News offers patients, patient advocates, and the general public the latest information about pain research, treatment, and care to guide their advocacy efforts and move the conversation forward.